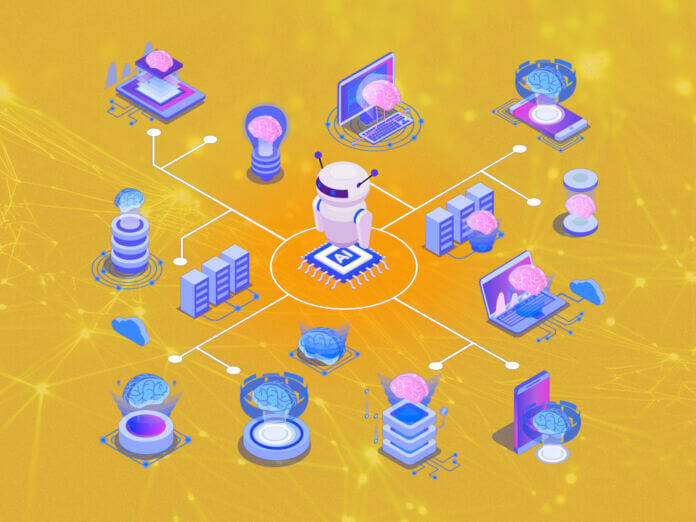
As of 2023, over 19% of all Internet of Things (IoT) deployments comprised over 10,000 devices. As the global mass deployment of IoT devices increases, so does the abundance of data it generates, leading to the emergence of new applications of AI now, and in the future.
The Intersection of AI and IoT
This convergence of AI and IoT is an accelerating trend as synergies between them come naturally. Essentially, IoT fleets generate a large amount of data. Big data is the fuel for AI machines to learn from and make intelligent decisions without human intervention.
AI-powered IoT enables businesses to gather real-time information, providing valuable insights for more accurate decision-making. As a result, they can reap a series of benefits, including automating repetitive tasks, optimizing energy consumption, maximizing cost efficiencies, analyzing customer preferences to design personalized experiences, and much more.
Let’s not forget about cyber security. Because of their nature, IoT devices are prone to malicious attacks. Well-designed software can exploit a single weak device to infiltrate entire networks.
Below, we outline how AI can prevent bad actors from infiltrating your network, alongside some of the most common applications of AI in the IoT ecosystem.
Enhancing Anomaly Detection with AI and IoT
Currently, the most common and main role of AI in IoT is anomaly detection. Anomalies can come in many different forms and are essentially deviations from normal device or network behavior.
A common use for AI algorithms is to detect unusual behaviors. These may indicate a malfunction in a device, or even an all-out cyber-attack, helping to raise alarms and inform businesses when these scenarios occur. Allowing them to take a more proactive approach toward cybersecurity and general device maintenance.
One example extends to time series forecasting which can be utilized for anomaly detection. Time series forecasting involves building models through historical analysis and using them to make observations to drive future decision-making.
For IoT devices and fleets, historical network activity and signals can be monitored and analyzed to detect unexpected behaviors. This behavior extends to suspicious scenarios where there is an overabundance or complete lack of network activity.
Leveraging Device Profiling
An additional use for the time series signal analysis is profiling the devices. Device profiling will allow businesses to group their devices into clusters according to their similar daily network activity profile for streamlined management.
This practice is most used for user and purpose recognition, based on the individual user or activity fingerprint in the profile.
However, activity profiling can also help to detect which devices are functional, vulnerable, or require security updates. It also provides an alternative approach to detect suspicious activity. Any deviation from the expected daily profile will be considered an attack.
Lastly, profiling can also serve as a classification tool for the attack. Common IoT devices and network attacks come from the same source and tend to be designed in similar ways. This leaves a trace in the activity profile, which opens the door for signature-based intrusion detection.
As the AI algorithm learns from previous attacks on IoT devices, businesses can design a way to recognize and react faster to new incoming intrusions.
Text Classification
This concept is rooted in language processing that assigns a set of predefined categories to open-ended texts. Text classifiers can organize, structure, and categorize text such as documents, transcripts, and more.
In the IoT ecosystem, language processing is mainly used for question-answering systems, voice recognition, and interpreting natural language commands (voice-activated control). Another use is analyzing social media content, like sentiment analysis of posts surrounding a particular product to further tailor specific content to end-users.
While this application remains in its infancy in IoT, possible uses may emerge as both AI and the IoT ecosystem continues to mature.
Optimization
A common use of AI in mass deployments of IoT is for optimization and better resource allocation. Such use can result in cost efficiencies which save businesses considerable time and money.
For instance, AI in IoT could be useful in a supply chain and logistics company with tens of thousands of IoT devices deployed around the world with a variety of use cases. Intermittent battery recharging across this huge network of thousands of connected devices can be improved with AI.
Also, the company can integrate a system to measure and regulate fuel efficiency. As gas prices continue to rise, fuel efficiency and route optimization are vital.
In this case, implementing feedback mechanisms with optimization algorithms in software can ensure the company is minimizing fuel consumption through optimal route planning.
Automation
Finally, autonomous decision-making is becoming a more mainstream application in IoT as well, especially in smart home systems.
This is because managing a series of different IoT devices can be challenging for end users who are not equipped with professional technical knowledge. Automating device management and enabling automatic responses to users’ needs helps to overcome these obstacles.
For smart utilities, automation systems for lighting and heating can even predict and optimize energy usage to help end users save money. It can also monitor excessive electricity use, recognize the issue, and rectify it without any human intervention or technical knowledge necessary.
Closing Thoughts on AI and IoT
The integration of AI with IoT helps to build smart, secure, and sustainable environments.
On one hand, IoT automates and optimizes real-world processes and reduces the need for direct human participation. However, it doesn’t eliminate the need for human judgment and decisions.
This is where AI can step in and unlock the full value of IoT systems. Integrating AI in IoT brings tangible benefits and insights to businesses and users alike to reduce complexity and enable better resource management.